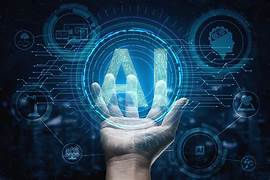
BY JOE PROCOPIO, FOUNDER, TEACHINGSTARTUP.COM@JPROCO
Can almost anyone be successful with A.I.?
That depends.
Can almost anyone learn to code?
Wait. Don't answer that yet. Because I don't want to get into a debate about coding skills. Not yet. What I'd rather talk about are the soft skills you need to be successful with A.I. (or in spite of it), and how they have little to do with writing code.
Hiring an army of natural language generators
I was a part of one of the first VC-backed natural language generation (generative A.I.) successes, Automated Insights, starting back in 2010. I invented the part of our platform and ran the side of the house that brought the A.I. skills that weren't code-specific.
Now, to be transparent, I am a developer. But I was tasked with hiring a small army of people who could provide the knowledge to be able to turn data into words -- words that had more meaning than just looking at the data itself.
In other words, I had to find people who could not just use A.I., but could succeed with A.I.
Back in 2010, when NLG was a new thing, even before it had a name, I had no idea what those necessary skills might be, and it took a lot of trial and error to figure it out.
Why these skills are important
I'm a big proponent of no-code and low-code. One of the arguments I make to support the no-code movement, and one that gets me into trouble with "real" coders, is that all of us, myself included, are just manipulating someone else's lower-level code.
I mean, it's 2023. Very few of us are writing low-level machine language. We're superusers. Really super users.
Oh, so, can almost anyone learn to code? Meh. Sure. Depends on how deep you want to get into the syntax.
But what separates great coders from terrible coders is a set of skills that has nothing to do with memorizing syntax. What makes great code elegant, reusable, and efficient are the soft skills that develop elegant, reusable, and efficient solutions that beg to be brought into reality by writing great code.
Over the past few years, no-code and low-code have evolved into something that looks a lot like a visual replacement for textual coding syntax. And since it continues to evolve at a breakneck pace, it's quickly catching up to textual syntax.
Will no-code ever replace real code?
Who cares?
Let's instead talk about the underlying skills you need to be great at no-code, real code, or A.I.
The skills that go beyond code
OK. So let's state the obvious for the purists. You still need code to implement the solutions these skills produce, whether it's code you learn on your own at a lower level, or no-code you implement at a higher level.
Now, if great code comes from a great solution, a great solution comes from great problem-solving. But I'm not going to be lazy and leave it at that. I solve a hundred problems a day and very few of them have to do with coding. Not zero, but very few.
So here are the problem-solving skills you need to develop the great solutions that become the great code that leads to successful A.I.
Understand how data impacts the problem
This also seems painfully simple, but there are a number of questions that are easy to answer without having to understand why:
What's the problem you're trying to solve, along with its adjacent problems?
Who else is trying to solve those problems and how?
Exactly how much of those problems does your data solve and how well?
How does your company sell and profit from that solution?
Automated Insights started exclusively in the sports industry. My first lesson was that people who understood exactly how data solved problems in the sports business were far more likely to succeed than either experienced data scientists or nontechnical sports industry veterans.
Application of business theory, product theory, and customer theory
What happens when you put a bunch of machine learning specialists, data scientists, and technologists in a room?
That's not a joke. We did it. Turns out the answer is absolutely nothing.
I'm being somewhat facetious. Once we had some traction in the market, I put together an innovation team to think about the science of A.I. and where we should head next. A lot of crazy ideas came out of that room -- a lot of fun, crazy, brilliant ideas. But none of them were rooted in business, and none of them could be effectively MVP'd, let alone fully realized.
Science is awesome. But what you build in a lab usually stays in the lab without a lot of heavy lifting. Instead, we relied on people who understood how to build amazing products, could bring them to market fast, and wanted to make customers successful.
Creativity focus and the application of ideas
Everyone thinks they're creative. Everyone thinks they have amazing ideas. And you know what? They're right.
But that doesn't mean anything if the creativity isn't focused and the ideas aren't applied. And usually, almost always, the only way to get better at either of those skills is by trial and failure.
We rolled out a few versions of our product at Automated Insights before we found the application of our idea that stuck. Today, I'm on the third iteration of one of my more recent projects after the first two -- same creative foundation, same idea but executed poorly -- failed.
Pattern recognition and trendspotting
If there's a reason I'd call myself a good coder, or good with A.I., this would be it.
We're in the populist stage of generative A.I. where we're seeing a lot of ChatGPT-style data in the wild that's been generated just for the sake of regurgitation of data.
The real skills aren't in spitting back data as words, something I forbid at Automated Insights. Instead, the hard part of what we did was figuring out meaningful trends, deltas, and milestones within that data. And while any budding data scientist can write formulas to detect or mimic that behavior, being able to visualize patterns, realize trends, and find milestones that have meaning is a soft skill that is essential for producing useful A.I. output.
What happens next -- developing state machines
This is the most developer-like skill you must have.
The best A.I. use cases don't just tell you what happened, they also tell you what should happen next. In traditional coding, you wrangle this with a state machine, represented as a flowchart-style diagram that captures the if-this-then-that nature of what computers do -- or "how they think."
This was the very foundation of our NLG platform. And by the time we were done, we had a multidimensional array of these state machines that made the decisions that generated useful, insightful, and valuable content.
If you can visualize, document, and implement state machines, you're well on your way to figuring out how to make the multitude of complex and related decisions that our human brains are making perpetually.
And that's what A.I. is really doing underneath all that code.
Commentaires